What Is Generative AI?
Generative AI can glean insights from existing data to create fresh and authentic content on a large scale. This technology preserves the essence of the training data while avoiding direct replication. It can generate diverse innovative content, including images, videos, music, speech, text, software code, and product designs.
Various techniques are employed within generative AI, which is constantly evolving to enhance its capabilities. AI foundation models hold prominence. These models undergo training on extensive sets of unlabeled data, enabling adaptability across different tasks through additional fine-tuning. Developing these models necessitates sophisticated mathematical algorithms and substantial computational power, and they function as predictive algorithms.
Today, generative AI most commonly creates content in response to natural language requests—it doesn’t require knowledge of or entering code. However, the enterprise use cases are numerous and include innovations in drug and chip design and material science development.
What’s Behind the Sudden Hype About Generative AI?
The sudden surge in hype surrounding generative AI can be attributed to several factors. Gartner has been closely monitoring the progress of generative AI since 2020, including tracking it on its Hype Cycle™ for Artificial Intelligence and recognizing it as one of the Top Strategic Technology Trends for 2022. Over time, generative AI has transitioned from the Innovation Trigger phase to the Peak of Inflated Expectations.
However, generative AI captured mainstream attention in late 2022 with the launch of ChatGPT. a chatbot developed by OpenAI capable of remarkably human-like interactions. This breakthrough innovation garnered widespread popularity virtually overnight and drew significant public interest. Similarly, OpenAI’s DALL·E 2 tool, which generates images from text, contributed to the excitement surrounding generative AI.
Gartner envisions generative AI evolving into a general-purpose technology with a transformative impact akin to previous groundbreaking technologies such as the steam engine, electricity, and the internet. While the initial hype may diminish as the realities of implementation become apparent. The long-term impact of generative AI is expected to grow significantly as individuals and enterprises continue to explore and discover innovative applications for the technology in their daily work and lives.
Benefits of Generative AI
A subset of Artificial Intelligence has gained significant attention and recognition for its numerous benefits and diverse applications across various domains. Here are some of the key advantages and applications of generative AI:
Content Generation:
Generative AI models can produce diverse forms of content, including text, images, videos, and music. This capability is particularly useful in creative industries such as marketing, advertising, entertainment, and design, where generating engaging and personalized content at scale is essential.
Data Augmentation:
Generative models can be used to create synthetic data, which can then be used to augment training datasets for machine learning models. This approach helps improve the robustness and generalization of machine learning algorithms. Especially in scenarios where collecting large amounts of real-world data is challenging or expensive.
Creative Tools and Assistance:
These systems can be creative tools and assistants for artists, designers, writers, and other creative professionals. These systems can generate novel ideas, assist in the creation process, and provide inspiration by generating variations of existing concepts.
Personalized Recommendations:
Generative models can analyze large volumes of user data to generate customized recommendations in various domains. Which includes e-commerce, entertainment, and content consumption. By understanding individual preferences and behavior patterns, these systems can offer tailored suggestions that enhance user experiences and increase engagement.
Natural Language Understanding and Generation:
Generative AI models have advanced natural language processing capabilities, enabling them to understand and generate human-like text. These models can be used for language translation, summarization, question answering, dialogue generation, and content generation.
Medical Imaging and Diagnosis:
In healthcare, generative AI models are being utilized for medical imaging analysis and diagnosis. These models can generate high-resolution images, and enhance low-quality images. Also, assist medical professionals in detecting abnormalities and diagnosing diseases from medical scans such as MRI and CT scans.
Drug Discovery and Material Design:
Generative AI techniques are increasingly being applied in drug discovery and material design. These models can generate novel molecular structures with desired properties. That accelerates drug discovery and optimizes the design of materials for various applications, including pharmaceuticals, energy storage, and electronics.
Virtual Reality and Simulation:
Generative AI can generate realistic virtual environments and simulations for training, entertainment, and research purposes. These systems can generate virtual scenes, characters, and objects, thus enabling immersive experiences in virtual reality (VR) applications and facilitating simulation-based training in fields such as aviation, healthcare, and manufacturing.
Fraud Detection and Cybersecurity:
Generative AI models can analyze data patterns to detect anomalies and identify fraudulent activities in various domains, including finance, e-commerce, and cybersecurity. By continuously learning from new data and adapting to evolving threats, these systems can enhance the security and integrity of digital systems and transactions.
Robotics and Autonomous Systems:
Generative AI techniques play a crucial role in robotics and autonomous systems by enabling robots to perceive and interact with their environment effectively. These models can generate representations of the world, plan and execute actions, and learn from experience to improve performance in tasks such as navigation, manipulation, and object recognition.
Overall, generative AI offers a wide range of benefits and applications across diverse domains. It empowers organizations and individuals to create, innovate, and solve complex problems in new and exciting ways.
What Are the Risks of Generative AI?
The risks associated with generative AI are substantial and evolving rapidly. Various threat actors have already exploited the technology to create “deep fakes” or counterfeit products and to perpetrate increasingly sophisticated scams.

ChatGPT and other tools have undergone training on vast amounts of publicly available data. However, they haven’t been designed to comply with the General Data Protection Regulation (GDPR) and other copyright laws. Which makes it crucial to carefully consider your enterprise’s use of such platforms.
Key oversight risks to monitor include:
Lack of Transparency:
Generative AI and ChatGPT models are unpredictable, and even the companies developing them may need to understand all aspects of their functioning fully.
Accuracy:
Generative AI systems may generate inaccurate or fabricated responses. Before relying on or publicly disseminating information derived from them. It’s essential to assess all outputs for accuracy, appropriateness, and practical usefulness.
Bias:
Establish policies or controls to detect biased outputs and handle them according to company policy and relevant legal requirements.
Intellectual Property (IP) and Copyright:
There are no verifiable assurances regarding data governance and protection concerning confidential enterprise information. Users should assume that any data or queries entered into ChatGPT and similar platforms may become public information. Enterprises should implement controls to prevent accidental exposure of IP.
Cybersecurity and Fraud:
Malicious actors may exploit generative AI systems for cyber and fraud attacks, including using deep fakes for social engineering purposes. To assess coverage for AI-related breaches, it’s essential to implement mitigating controls and confer with cyber-insurance providers.
Sustainability:
Generative AI consumes significant amounts of electricity. Choosing vendors prioritizing reduced power consumption and utilizing high-quality renewable energy can help mitigate the impact on sustainability goals.
Addressing these risks requires careful consideration and proactive measures to ensure the responsible and secure use of generative AI technologies within enterprises.
What are the Challenges of Generative AI?
As an evolving field, generative models are still in their nascent stages, leaving ample room for growth in several areas:
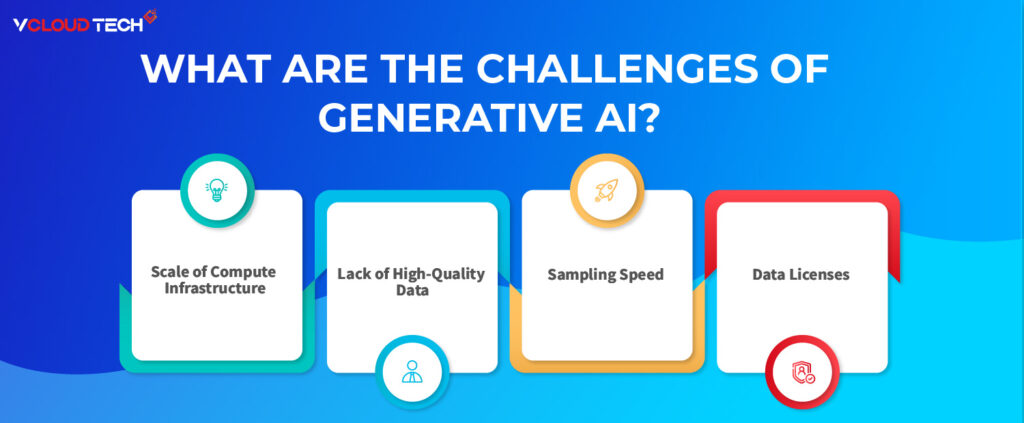
Scale of Compute Infrastructure:
Generative AI models often boast billions of parameters; additionally, they necessitate fast and efficient data pipelines for training. Substantial capital investment, technical proficiency, and large-scale computing infrastructure are imperative; moreover, for maintaining and advancing generative models. For instance, diffusion models may require millions or even billions of images for training. Consequently, training such vast datasets also demands massive computational power, necessitating AI practitioners to procure and effectively utilize hundreds of GPUs.
Sampling Speed:
Given the generative model scale, generating instances can be latency. This is particularly crucial for interactive scenarios like chatbots. AI voice assistants, or customer service applications, where immediate and accurate responses are essential. As diffusion models gain popularity for their ability to produce high-quality samples, their slow sampling speeds become increasingly apparent.
Lack of High-Quality Data:
Many utilize generative AI models to generate synthetic data for various purposes. However, despite generating vast amounts of data worldwide daily, not all of it is suitable for training AI models. Additionally, generative models require high-quality, unbiased data to function effectively. Furthermore, certain domains need more data for model training. For example, there are limited 3D assets available, and their development is costly. Addressing these gaps will demand substantial resources to evolve and mature.
Data Licenses:
Many organizations encounter difficulties obtaining commercial licenses for existing datasets. Also constructing custom datasets for training generative models, which may add to the data quality challenge. This process is critical in preventing intellectual property infringement and requires careful consideration.
Numerous companies, including NVIDIA, Cohere, and Microsoft, aim to support generative AI models’ ongoing growth and advancement. Additionally, they may offer services and tools to address these challenges. Furthermore, these products and platforms abstract away the complexities of scaling up and running models.
How Will Generative AI Impact the Future of Work?
Generative AI is poised to impact the future of work across various industries profoundly. Moreover, as businesses increasingly rely on generative AI for content creation, the roles of many individuals who traditionally engage in content creation will undergo significant transformation. Consequently, these individuals will transition into roles as content editors, requiring a new skill set to curate and refine AI-generated content effectively.
Furthermore, integrating generative AI into applications will reshape how the workforce interacts with technology. Moreover, applications will become more conversational, proactive, and interactive, necessitating a redesigned user experience. Additionally, in the near term, generative AI models will evolve beyond merely responding to natural language queries to actively suggesting content or solutions that the user may not have explicitly requested. For instance, a request for a data-driven bar chart might prompt the AI to offer alternative graphics that it deems relevant. While this has the potential to enhance worker productivity, it also challenges traditional notions of human leadership in strategy development.
Moreover, the overall impact on the workforce will vary significantly depending on factors such as industry, geographical location, company size, and the nature of its offerings. However, this will substantially change job roles, skill requirements, and how individuals engage with technology in the workplace.
Reach out to us and book a Free Consultation with vCloud Tech or chat with one of our representatives. Connect with us on Twitter, Facebook, Instagram, and LinkedIn for more information.